Expert Consultancy
We provide expert consultancy in all stages of the data analytics lifecycle from helping to define the business need, skill sets and data resources to the application of machine learning models and deployment of the final predictive analytics solution to effect the desired outcome.
Our consultancy service can be described according to the following two themes:
1. Purpose, Strategy and Process for Effective Predictive Analytics
Defining and understanding the purpose of your analytics journey is the critical starting point for any successful analytics project. It is common that an organisation will want to define clear external value for their projects, typically referring to growth of some form. Depending on the organisation, this might be: sales growth by reducing customer churn or more targeted advertising and pricing; increased efficiency in the manufacturing process resulting in reduced production costs and thus increased profitability; increasing the availability of hospital beds within Accident and Emergency departments through more accurate classification of existing patients that can be safely discharged sooner (based on their illness and pattern of vital signs); local governments increase road safety by more accurately identifying accident hot spots and making appropriate interventions such as increased traffic control.
Once the purpose or project goal has been unambiguously defined, the strategic roadmap must be developed and organisational capacity established in order to successfully achieve it. Typically this will involve agreeing a systematic process, such as the CRISP-DM methodology or similar and reviewing the existing IT infrastructure, and administrative and technical knowledge and skill sets within the organisation with a view to identifying skill gaps and risks.
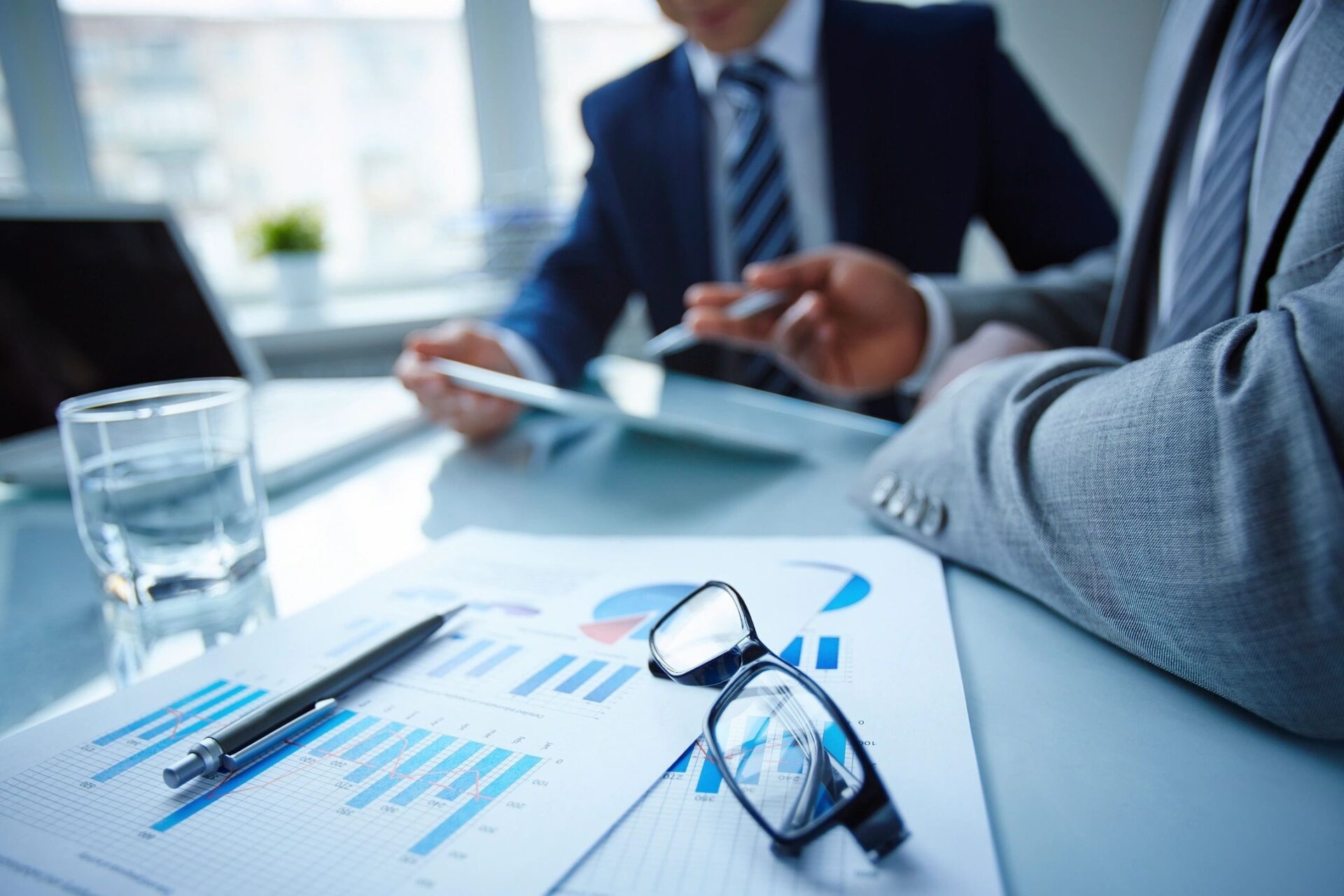
We will work effectively with key stakeholders to advise and facilitate you in one or more of the following areas:
- establish predictive analytics projects that add external value to your organisation;
- establish a standard reproducible approach for your predictive analytics projects (that have well-defined phases, steps, techniques, tools and deliverables);
- perform a root and branch review of your relevant organisational processes and practices to identify and alleviate any barriers or bottlenecks to the successful completion of your projects;
- successfully manage your analytics projects and associated change management processes to ensure effective deployment of your analytic solutions.
2. Technologies, Resources and Skill Sets
A systematic review of your current practices, processes and organisational roles in relation to data processing and analytics will help to reveal key enablers and obstacles to successful predictive analytics. The key enablers refer to the inspiring leadership, smart funding, metrics / key performance indicators, IT infrastructure for effective data warehousing, and innovative interdisciplinary teams analysing departmental and enterprise-level data to gain insight and deliver change that adds value. Conversely, there are numerous reasons why predictive analytics projects fail. According to the recent whitepaper, Data Modernization by Rockspace Technology, the four most common reasons are: data discovery challenges (poor access to high quality data), excessive cost (caused by inflexible data infrastructure and expensive talent), complexity (a mix of structured data, suitable for relational databases, and large volumes of unstructured data such as tweets, images, videos and audio), and skill gaps (lack of in-house skill sets to optimise and exploit their data architecture and analytics eg Data Architects, Data Scientists, Machine Learning Engineers, Full Stack Developers).
To facilitate you to remove overt or latent barriers to the success of your predictive analytics projects, we can provide expert consultancy in the following areas:
- data veracity and cost analysis (from point of entry into transaction process systems, through the Extract-Transform-Load layer and to the resulting application layer where data is analysed using OLAP cubes, Dashboards);
- data variety (review of the available structured and unstructured data across enterprise databases, how it is being processed and used for analytics with a view to identifying more efficient and effective methods of integration and mining for insight);
- technology infrastructure (cost-benefits analysis of existing platforms used for data warehousing and analytics such as local solutions like Microsoft SQL Server, SAP, Microsoft SQL Server Analysis Services, Microsoft Power BI or cloud-based solutions such as Oracle’s Autonomous Data Warehouse, Amazon Web Services, Microsoft Azure or Google Cloud);
- talent (review of existing knowledge and skills set in database design and administration, data science and analytics to identify skills gaps and candidate solutions);
- machine learning solutions (we have significant expertise in the use of cutting-edge machine learning techniques such as decision tree learning, regression analysis and artificial neural networks / deep learning for a variety of problem domains requiring classification, prediction or time-series forecasting);
- enhanced governance to enable optimum engagement between multi-disciplinary teams through standardised practices and accountability (eg RACI principles).